Why computational neuroscience and AI will converge
Outline
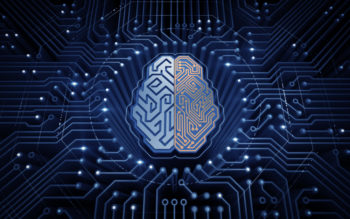
Neural networks have peaked in their ability to deliver effective and meaningful results. There are four big developments that will happen with AI. A crossover with computational neuroscience will happen soon. What will be the impact of this crossover? There will be an increasing focus on how AI algorithms interact with one another
The limitations of neural networks
Today neural networks dominate the landscape of AI and AIOps, but I've said many times that this is unsustainable. Neural networks have peaked in their ability to deliver effective and meaningful results. The science has issues with basic intractability, mismatch and inherent latency. Even though there is a lot of investment in neural networks, it's bearing on AIOps and the real-time business community is limited. Which brings me on to computational neuroscience, which I believe will benefit AI enormously.As I gaze into the future in terms of how AI is likely to evolve, I expect there to be a lot of crossover with computational neuroscience. What's happening at the moment with neural networks is an early attempt to cross fertilize with AI, but this is failing and will continue to do so.
What is computational neuroscience
It's an attempt to take the complex and poorly understood behaviours of the human brain and associated nervous system and develop both mathematical and algorithmic models to try to understand their behaviour. You can compare computational neuroscience to economics or climate science. In all of these cases you have an immensely complex system with visible, but poorly understood, contours. We hope we can learn something about these systems to make high level predictions, which is achieved by building computational models that are either straight algorithms or a set of mathematical equations to try and get some insight into these large complex systems. This approach is entirely different from other scientific endeavours such as physics and chemistry, where you start with well defined behaviour and then try to build from the bottom up to understand, for example, why atoms behave the way they do, or how molecules or cells interact. Think of computational neuroscience, economics, and climate science as top down sciences, as opposed to classical bottom up sciences. Generally, computational neuroscience will give you some indication as to how the brain and nervous system works.When you look at it that way, one of the things that becomes very interesting is that AI and computational neuroscience have many similarities. However, there is a perception that there is a massive difference between the two disciplines, many perceive computational neuroscience as a bottom up science and see AI as an engineering project. That is wrong, both of them are top down sciences that are investigating very similar and heavily overlapping domains. Therefore, in the next five to ten years we are going to see more crossovers between the two disciplines
What will be the impact of this crossover
Firstly, there will be an increasing focus on how AI algorithms interact with one another. I think in most academic research and industrial efforts there is a lot of emphasis on developing and working with individual algorithms, but there is very little attention given to how the collection of algorithms interact with one another from an architectural perspective. One of the reasons why is because we naturally think of intelligence as a space that co-exists and there is no interacting structure. The truth is that algorithms need to be carefully choreographed with one another. This is very evident in the field of AIOps and how the Moogsoft platform has evolved. We have different types of algorithms which function at different times and hand off their results to one another. The result is very similar to the architecture off the human brain as we understand it.As AI is deployed more systematically across more systems, the need to choreograph the interactions between the different algorithms will become more pressing. There is a vast body of knowledge which already exists on how, for example, visual systems interact with higher level conceptual categorization systems or how visual and auditory systems interact with one another. Therefore, it would be natural to look at the architecture of the brain as a starting point to design an optimal architecture for the interaction of various AI algorithms.
The inevitability of distribution
Secondly, AI research and industrial deployments has always focussed on centralized AI algorithms. In general, there is a drive to pull data in from various parts of the environment and take it to a single place where the AI algorithm is applied to it. I think increasingly there will be a focus on distributing algorithms geographically.If you look at the way cognitive processes are enacted in the brain, and especially in the nervous system, it is evident it can become a model for how intelligence can be modularized and distributed - not only conceptually but physically across a system. I think the way in which models are being developed on the computational neuroscience side to reflect distribution of intelligence will end up being a body of teachings for AI. To be fair, even in the field of computational neuroscience there has been insufficient focus on the need to modularize and distribute algorithms...but it's definitely coming.
The role of robotics
Thirdly, as industry becomes more and more interested in robotics (the application of AI to automation) there will be an increased focus on how intelligence and AI algorithms interact with physical world processes. So, as robotics moves from being theoretical to a genuine industrial endeavour, the models that have been built to understand how the brain interacts with the nervous system and the external world will play an increasing role in the advancement of AI.The importance of the cognitive process
Lastly, when we talk about machine learning or neural networks the focus is very much on the learning that takes place within an individual algorithm. It is not focused on how an entire system of algorithms evolves. As AI begins to recognize the importance of architecture and the choreography of algorithms; as it becomes more focused on distributed intelligence; as it becomes more focused on interacting with the external world; then I think we're going to develop systems whose entire cognitive apparatus evolves and learns with time.Computational neuroscience has absorbed and modified work conducted around cognitive psychology which has been embraced by the neural science world. I think this research has a lot to teach AI around the cognitive architectures it seeks to deploy in the industrial world.